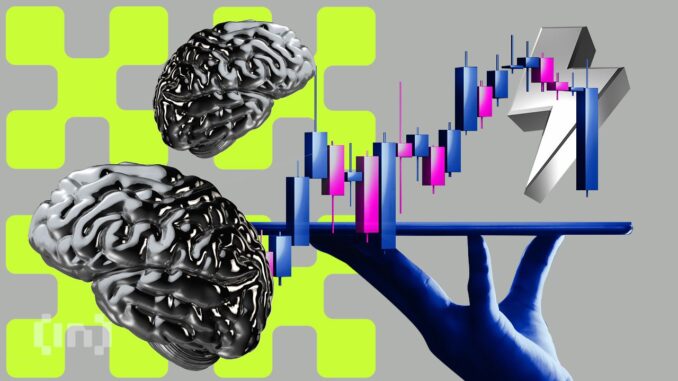
Exploring the environmental implications of Artificial Intelligence (AI) presents a Herculean task. This exploration forces a reassessment of the broader repercussions of our unyielding quest for innovation.
Every technological advance leaves behind an ecological footprint, often overlooked. This article ventures into the under-recognized environmental effects of generative AI tools.
The Unseen Consequences of AI: An Environmental Impact Assessment
Artificial Intelligence has quickly morphed into a potent force of transformation. Its influence now permeates diverse sectors like finance, healthcare, and entertainment.
However, beneath this innovative luster lurks an unheralded consequence: a substantial carbon footprint.
The sobering reality of the environmental cost tied to the burgeoning AI industry becomes palpably evident when focusing on energy-intensive generative AI tools.
AI’s Environmental Dilemma: The Carbon Footprint Conundrum
The energy consumption of AI is colossal. Training generative models such as OpenAI’s GPT-4 necessitates an extensive computational resource allocation. It extends beyond merely energizing servers with electricity.
Maintaining an ideal environment for these machines through physical infrastructure also consumes power.
For instance, cooling systems for servers and data centers notably escalate energy consumption, leading to considerable carbon emissions.
Compared to traditional digital activities, AI’s power usage is astonishingly vast. Training a single large-scale AI model can emit as much carbon as five cars throughout their lifetimes, including manufacturing.
This alarming fact gains further gravity when forecasting AI tools to become as prevalent as search engines, potentially causing energy expenditure and environmental impact to soar.
Deciphering the Energy Demands: The Complexities of AI Models
The substantial energy consumption of these brute-force statistical models originates from several characteristics:
Need for vast training examples: To accurately identify an object, for example, a cat, a model requires countless examples. These encompass images of cats from diverse angles, breeds, colors, shadings, and poses. Given the limitless possible representations of cats, the model must train on a wide array of cat variations to excel at identifying a novel cat.
Multiple training cycles required: The training process entails the model learning from its errors. If the model mistakenly labels a cat as a raccoon, it adjusts its parameters, reclassifies the image correctly, and retrains itself. This iterative process requires a significant number of training cycles.
Retraining required for new information: The model requires fresh training when encountering a new category, such as cartoon cats. It has to include diverse examples of cartoon cats, like blue or red ones, in the training set and begin training anew. The model lacks the capability for incremental learning.
Numerous weights and intensive computation: A standard neural network comprises many connections or weights represented by matrices. The network must perform multiple matrix multiplications across successive layers to compute an output until a recognizable pattern emerges. A single layer often demands millions of computational steps, and a typical network could contain dozens to hundreds of layers, resulting in energy-intensive computations.
Accessibility: A Double-Edged Sword
The escalating accessibility and incorporation of AI tools in mobile applications introduce a convoluted issue. While the ubiquity democratizes AI, it also amplifies the environmental impact.
As more people employ AI features daily, the energy demand for AI computations progressively grows.
Yet, there is no need for despair. The AI industry is beginning to acknowledge these environmental challenges. Researchers are striving to develop more energy-efficient AI models.
The objective is to accomplish more with less—preserve AI capabilities while reducing the energy required for computations.
Harnessing Renewable Energy: Paving the Way for a Greener Future
A growing trend of utilizing renewable energy sources in AI computation is emerging. Deploying solar and wind power to run AI operations can reduce carbon footprint significantly.
Tech giants like Google spearhead this movement, pledging to power all their operations, including data centers, with renewable energy. This pivot towards green data centers signals a promising progression toward a sustainable AI future.
Crafting a truly sustainable AI future poses a daunting challenge. The obstacles span from creating energy-efficient models to powering computations with renewable energy. Regardless of these hurdles, this journey remains indispensable.
The potential advantages of AI are too great to disregard, just as the environmental costs are too significant to ignore. As the AI revolution progresses, securing our planet’s future becomes paramount.
Towards a World of Enlightened Decisions
Recognizing AI’s transformative power must go hand in hand with grappling with its environmental toll. This acknowledgment should inspire action, leading to the striking of a delicate balance.
Efforts must go beyond just crafting intelligent machines to fostering a sustainable future. This involves promoting the development of energy-efficient AI models, advocating for renewable energy in AI operations, and nurturing a culture of sustainability within the industry.
These measures aren’t merely options but essential components of our path toward progress.
Riding the Wave: Efficient Model Architecture
Globally, researchers are making considerable headway in creating more energy-efficient AI models. Innovative model architectures, such as Transformer models, are gaining traction.
These models enable computations to be more efficiently parallelized, allowing them to process more data in less time, thereby reducing energy consumption.
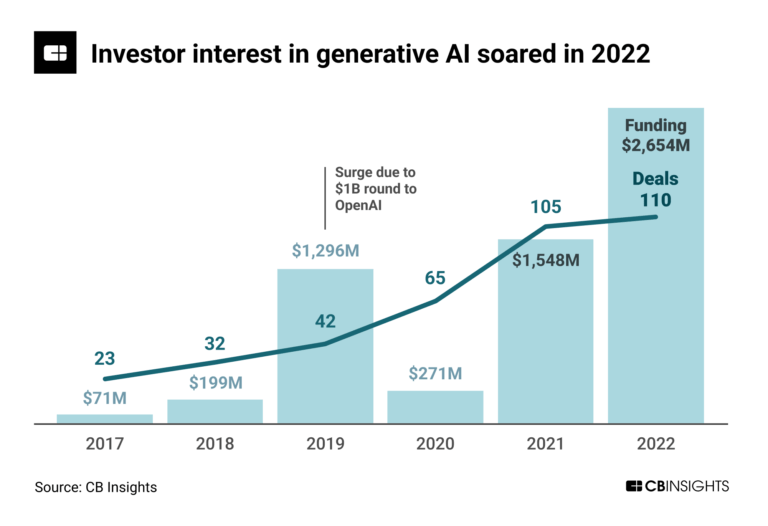
Moreover, businesses increasingly consider the environment in their AI operations. A movement towards greening data centers is underway, where these infrastructures are designed with minimal environmental impact in mind.
By employing efficient cooling methods, leveraging renewable energy, and strategically locating data centers in colder climates to decrease the need for cooling, significant progress is being made to mitigate AI’s environmental footprint.
The Crucial Role of Policy and Regulations
The influence of policy and regulations remains paramount. As seen in other industries, impactful change often requires collective action that transcends individual businesses.
Policies encouraging the use of renewable energy and establishing guidelines for energy-efficient practices can inspire industry-wide shifts toward sustainable AI.
Embracing the Challenge
The path toward sustainable AI is intricate and fraught with challenges. It’s an ongoing process demanding continual innovation, rigorous regulation, and a willingness to adapt. However, considering the enormous potential of AI, the effort is unquestionably worthwhile.
Now is the time to spotlight the less glamorous side of our digital revolution and grapple with its hidden environmental costs. Unveiling the environmental impact of AI and seeking sustainable solutions is more than just a matter of corporate responsibility. It’s a crucial step towards securing our planet’s and future generations’ health.
After all, the goal isn’t just to build smarter machines. The aim is to create a smarter, more responsible, and sustainable world. Let’s keep this mission at the forefront as we advance in the AI era. It’s not just the smart choice—it’s the only choice.
The post AI Unplugged: The Hidden Costs of Artificial Intelligence appeared first on BeInCrypto.
Be the first to comment